The unfolding AI Revolution presents a critical turning point for businesses, necessitating strategic adaptation or risk of obsolescence. Central to this adaptation is effective Data Governance. However, Gartner warns that a staggering 80% of companies stand to falter in scaling their digital business by 2025 if they fail to modernize their Data Governance approach. You can prevent this by following some Data Governance Best Practices, which we’ll explain in this article.
Executive Summary
Key challenges for effective Data Governance lie in data visibility, with 42% of organizational data often overlooked or unused (“dark”) despite extensive data collection. Isolated data silos and outmoded systems stymie data integration, while data security presents a considerable risk, evidenced by 83% of businesses experiencing at least one breach, incurring an average cost of $9.44 million per incident. Moreover, poor data quality, generating an average annual cost of $12.9 million, hampers effective decision-making. Confronting these challenges necessitates a shift towards a modern Data Governance strategy, accentuating collaborative data modeling, full-scale data observability, and streamlined data security procedures. Implementing such a proactive, federated approach to Data Governance guarantees data relevance, accuracy, and consistency while simplifying data protection complexities.
At Motius, we have supported many businesses through these transformations, enabling them to effectively harness data for the impending AI revolution and become genuinely data-driven entities, no matter where they currently reside in their Data Governance journey.
Update Your Data Governance or Perish
Revolutions mark the history of human progress. The Agricultural Revolution transformed us from nomadic hunter-gatherers to settled farmers – the Industrial Revolution mechanized production, leading to unprecedented economic growth. Today, we stand on the brink of another transformative era: the AI Revolution. This revolution, driven by data and artificial intelligence, reshapes every aspect of our lives and businesses. It’s been speculated about for decades under Industry 4.0, data-driven decision-making, data-driven companies, Medicine 3.0, etc. While we may not know the exact timeline or the duration of this transition, one thing is sure: it’s coming and coming sooner than we think.
As with previous revolutions, this transition presents a stark choice for companies: adapt your Data Governance or perish. Companies that need to leverage their data operationally and analytically will need help to survive. According to Gartner, through 2025, 80% of organizations seeking to scale digital business will fail because they need to take a modern approach to analytics and Data Governance. Recent breakthroughs in generative AI underscore the urgency of this transition. AI is coming, whether in your company or your competitor’s. About time for a (modernized) data strategy with effective best practices for Data Governance!
Four Pillars of Good Data Governance
The sheer amount of data can make feel trapped in a flood of information. But there are four crucial pillars for effective (digital) Data Governance that help you navigate the data jungle: data visibility, data integration, Data Governance, and data quality. If you manage to establish good Data Governance Best Practices in these four fields, you have a solid foundation for a data-driven (aka AI-driven) business.
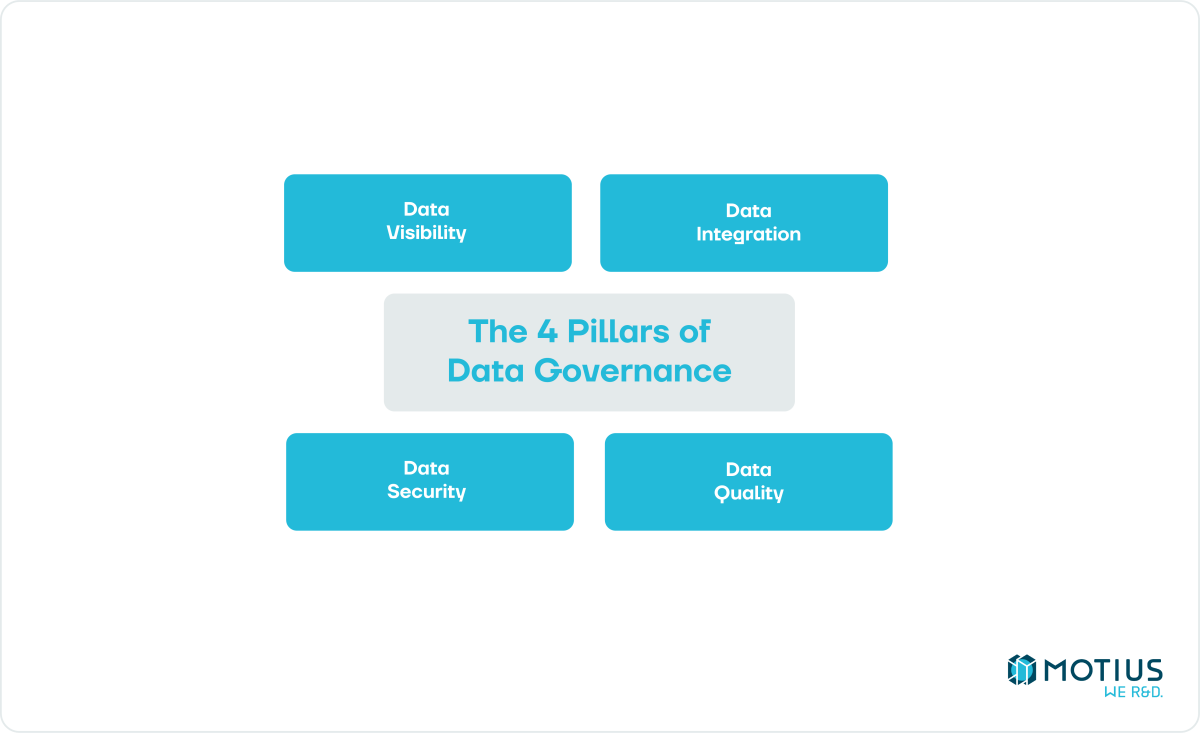
Data Visibility
The concept of data visibility pertains to the extent to which organizations can access, understand, and use their data. A major stumbling block for many companies is the existence of “dark data”. This is data that has been collected and stored but is not used for making decisions or generating insights, mainly because its presence is either unknown or forgotten. According to a data management provider Splunk report, 42% of respondents revealed that at least half of companies’ data was “dark”. This leads to missed opportunities for insight, an inability to make data-driven decisions, and unnecessary storage costs. The problem gets exacerbated as the size of the business grows – the larger the organization, the more data they generate, making data visibility even more difficult. Thus, effective Data Governance aims to increase data visibility and reduce such “dark data”.
Data Integration
Data integration involves the combination of data from different sources, providing a unified view that can be leveraged for insights. However, several obstacles often stand in the way. One significant barrier is data silos. These isolated data pools within an organization are not shared with other departments. Data silos hinder the flow of information, leading to disjointed insights and duplication of effort. Legacy data architectures also pose a significant problem. Often, they are rigid and not designed for the high level of integration required by modern businesses. The need for well-defined schemas for integrating different types of data adds to the complexity. In effect, data integration is a time-consuming task, requiring specialized technical resources. Make sure that Data Governance guidelines factor the necessary resources to integrate your data.
Data Security
In our increasingly data-driven world, data security is paramount. A lack of adequate data security and access management infrastructure can lead to breaches, significant financial losses, and damage to an organization’s reputation. Data governance plays a significant role in data security, ensuring that sensitive data is protected and that access is carefully controlled. Compliance with regulations such as the General Data Protection Regulation (GDPR) is also a key consideration, particularly for international businesses. However, the reality is sobering. According to an IBM report, 83% of organizations studied have experienced more than one data breach, with the average cost of a data breach in the US reaching $9.44 million. A significant risk that every contemporary Data Governance best practice should cover.
Data Quality
The final piece of the puzzle is data quality. It refers to the condition of a set of data values, which should ideally be consistent, accurate, and complete. However, businesses often need help with poor data quality characterized by discrepancies, inaccuracies, and gaps. A certain level of data quality is essential for AI applications. Therefore, every company that wants to use AI should embed quality standards in their Data Governance. But even without AI, data quality can have serious implications for the business. For instance, if data about customer preferences is accurate, a marketing strategy based on that data will likely succeed. According to Gartner, poor data quality costs businesses an average of $12.9 million annually, reflecting the high price of not getting it right.
Data Governance Best Practices 2.0
Now, all the explained cornerstones should be embedded in a good data strategy. What are then effective, concrete Data Governance Best Practices to achieve this? Ultimately, we aim to shorten the time from idea to insight. We want to use data to increase revenue and streamline operations. We need to understand that the most significant contributing factor to whether you will achieve that is how well your Data Governance works. In essence, dealing with the data jungle is challenging, and overcoming these challenges requires a paradigm shift in how we approach Data Governance. It calls for new strategies, technologies, and architectures that can help businesses navigate their way out of the jungle and into the clearings of valuable insights. A 2.0 version for Data Governance Best Practices.
The solution to this lies in a proactive federated Data Governance platform that is thought end-to-end and with data security at its core. Below, we outline a three-pronged approach to Data Governance, highlighting the importance of collaborative data modeling, end-to-end data observability, and simplifying data security operations.
Collaborative Data Modeling for High Data Quality
The first step towards efficient Data Governance involves establishing collaborative data modeling, ensuring a high degree of data quality. This approach involves the concerted effort of both business and technical users.
Business users define the use cases, document data points, and determine data quality metrics. Their role is crucial in ensuring the data collected and maintained is relevant, accurate, and usable for decision-making.
Technical users, on the other hand, focus on schema definitions, data types, relationships, and other data integrity checks. They create what can be termed a Data Contract, which guarantees a high level of quality and ensures data consistency across the organization.
Implementing a centralized repository for source schemas and metadata can further simplify data integration and exchange between data producers and consumers. Such a system enforces quality control and handles evolving data structures without breaking compatibility, thereby eliminating significant challenges associated with Data Governance.
End-to-End Data Observability Module
An end-to-end data observability module ensures complete visibility and control over data at every stage, from its origin to its use in analytics or decision-making. This Data Governance Best Practice consists of a three-pronged approach:
Mapping and documenting data lineage is a key part of this. It involves tracking and recording the origin and transformation flow of all data points. This creates a clear understanding of how data moves and changes within your systems, which is critical for data reliability, compliance, and troubleshooting.
Monitoring data reliability involves identifying patterns, trends, and anomalies in the data. Implementing mechanisms for this purpose helps maintain data quality and reliability by detecting potential issues such as inconsistencies and inaccuracies in data as well as compliance violations and privacy rule gaps.
Ensuring data integrity requires checks and validations to verify the accuracy and consistency of data. Detecting and addressing any inconsistencies or errors that may affect the reliability of your data is crucial for maintaining trust in your data.
Minimize Data Security Operational Complexity
Finally, simplifying data security operations can minimize the complexity associated with protecting sensitive data as well as managing data access and ownership across organizations. We recommend implementing this Data Governance Best Practice by:
- Centralized security governance: Establish a central point for creating and deploying access policies, and let the governance platform handle the integration and enforcement of these policies. This minimizes the complexity and likelihood of errors associated with manual policy management, or siloed data access controls.
- Adopting a federated ownership model: This model removes IT bottlenecks by giving data owners and stewards control over their shared data. It enables the definition of data access and privacy policies from their Data Governance workbench while adhering to the company’s global data protection policies.
These measures ensure a dynamic, secure, and efficient system for Data Governance, paving the way for organizations to leverage their data to its full potential in the era of the AI Revolution.
Let’s Discuss Data Governance
In the face of the AI Revolution, companies must take Data Governance seriously, as it is the biggest roadblock for most companies to become truly data-driven. By adopting a modern Data Governance Best Practices, companies can survive and thrive in this new era.
At Motius, we understand the challenges and nuances of Data Governance. We are deeply knowledgeable in this sphere and are committed to helping companies navigate the data jungle. Whether you’re just starting your journey or looking to improve your existing Data Governance practices, we’d love to discuss it with you. Let’s get in touch and start the conversation.